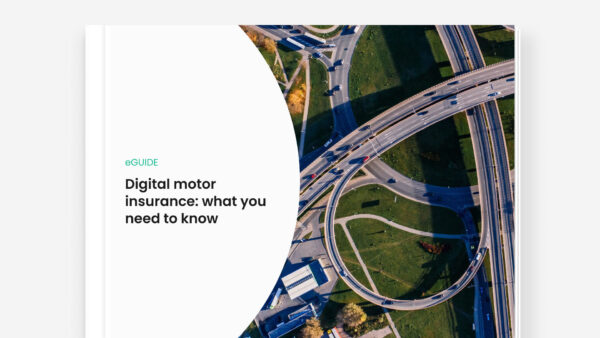
One of the basic principles of insurance is to spread risk. When a lot of people each take out an insurance policy and pay a premium to the insurer, that “pool” of money is used to pay for any losses that occur within the group.
This might sound an overly simplistic description of the insurance process. However, calculating how much everyone should pay towards the “pool” is a complex puzzle that insurers are still trying to solve. Fortunately, tech is proving to be the solution.
The traditional insurance model uses “proxies” such as where a person lives, their age, job, and vehicle model. To simplify – again – let’s imagine two people of the same age live on the same street, have the same job, and drive the same car. Although they may have a different “no claims” discount, and may each pay a different excess, these factors aside, they would pay the same basic premium. Yet one might be a safe driver and the other an unsafe driver, and the status of this would not necessarily be evident in claims history. The safe driver could effectively be subsidising the unsafe driver. Both premiums would need to be high enough to cover the cost of any losses, which would be more likely to come from the unsafe driver.
Enter telematics. Over the last decade, some insurers have refined their pricing model by offering policies with an element of mobile risk-based pricing. However, traditional telematics isn’t without its downsides. The cost is one major drawback for insurers who rely on a black box or ODB device to obtain telematics data. Another is the friction between drivers and suppliers. There is also scope for false negatives in methods of driver risk management that rely solely on driver “events”, as I discussed in a recent article.
Fairness matters in today’s insurance market. In 2021, we saw the introduction of the Financial Conduct Authority (FCA)’s “pricing remedy”. The outcome is that insurers can no longer offer a renewal price to an existing customer that is higher than the equivalent new business price for a new customer. This was the result of a market study that found home and motor insurers were identifying customers who were more likely to renew with them, then increasing the premium for those customers. The process was referred to as “price walking”. In summary, customers who stayed with insurers for longer were paying higher premiums to subsidize discounted policies for new customers.
Pricing is a complex and critical process. Insurers need to offer competitive prices at the same time as covering loss costs. A system that’s capable of attracting new customers while reducing loss ratios is required.
Not only does insurance pricing need to be fair, but it needs to be dynamic. This particularly matters to the younger generation, alongside the tech-savvy. Vehicle ownership models are changing. Spending habits are changing. The way customers buy insurance is changing. And insurers need to change if they expect to engage successfully with today’s consumers.
The future of insurance is all about fairness and personalization. Usage-based insurance is likely to grow in popularity, with drivers paying only for what they use. Behavior-based insurance is also likely to come into its own. This model enables drivers to be recognized for safer habits and pay according to their personal risk level.
In many ways, insurers are playing a guessing game when it comes to pricing. But, with the technology available today, there is no need to. Pattern AI that is interpreted into clear insights for the insurer enables them to get ahead of the competition. By leveraging the latest AI technology, insurers no longer need to rely solely on proxy factors to price risk. They can of course still set an initial rate using age old proxy ratings, then price accurately as they obtain mobile risk data. They could also however simply move to a “try before you buy” model.
Offering personalized insurance products priced according to an individual’s specific needs and behaviors is good for both consumer and insurer. Price is central in consumer decision making and a policy based on AI enables fair pricing for customers based on usage. For the insurer, it enables earlier risk forecasting of loss, more accurate risk management and therefore improved loss ratios. Overall, it enables insurance profitability.
At Greater Than, our pattern AI is based on a machine learning system trained on real vehicle data and pattern recognition. To date, it has identified over 7 billion different ways to drive a vehicle. These patterns, along with actual crash statistics, allow us to identify the risk of a traffic crash occurring.
Our pattern AI generally requires no additional in-vehicle technology. It can use the vehicle’s bluetooth and GPS data from a smartphone to enable insurers to better understand risk at an individual level. By having real-time data analysis to hand, insurers can see crash probability per driver and can then correlate this with the real cost of potential claims.
The winners in motor insurance will be those that utilize technology to provide fair, personalized, dynamic pricing and innovative propositions for the consumer. Pattern AI enables insurers to predict the profitability of the customers they acquire in a very short time frame and enables retention based on fair pricing. It also provides a uniform and comparable measurement of risk, regardless of vehicle type and geography. Together, these benefits provide an entirely new forecasting landscape for underwriters and risk managers.
Contact Greater Than or book a meeting with me to learn more about fair insurance pricing using AI.